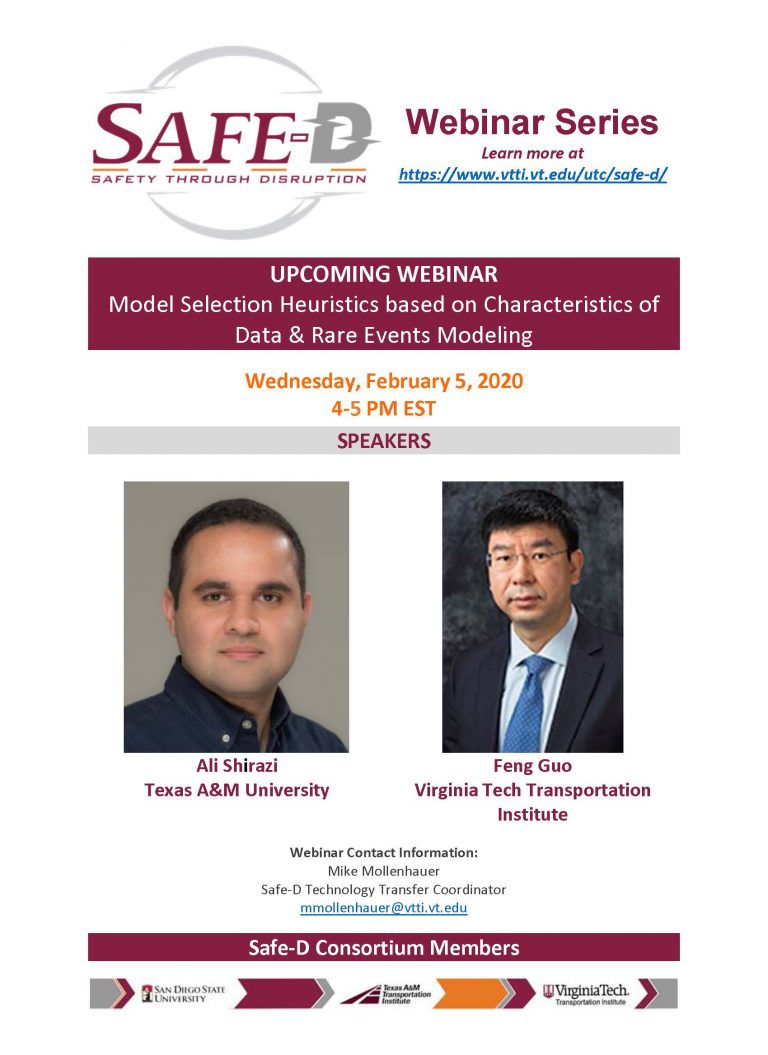
Please join us for the next webinar in the Safe-D Webinar Series, to be held February 5th from 4-5pm EST.
Upcoming Webinar: Model Selection Heuristics based on Characteristics of Data & Rare Events Modeling
Date: Wednesday, February 5, 2020
Time: 4-5 PM EST
Join the Webinar
Webinar Overview
Part 1: Model Selection Heuristics Based on Characteristics of Data. Transportation analysts usually employ post-modeling methods, such as Goodness-of-Fit statistics or Likelihood-based Ratio Tests for selecting the best distribution or model. These metrics require all competitive distributions or models to be fitted to the data before any comparisons can be accomplished. Given the continuous growth in introducing new statistical distributions, choosing the best one using such post-modeling methods is not a trivial task, especially given all theoretical or numerical issues the analyst may face during the analysis. Furthermore, and most importantly, these measures or tests do not provide any intuitions about why a specific distribution or model is preferred over another (Goodness-of-Logic). This presentation describes a methodology to design heuristics for Model Selection based on the characteristics of data, in terms of descriptive summary statistics, before the competitive models are fitted. The proposed methodology employs two analytic tools: (1) Monte-Carlo Simulations and (2) Machine Learning Classifiers, to design simple heuristics to predict the label of the ‘most-likely-true’ distribution for analyzing data.
Part 2: Rare Event Modeling. The rare event nature of crashes brings challenges in crash modeling and prediction. This study focuses on the following two aspects: 1) propose bias adjustment for more accurate estimation of the safety impact of a risk factor; 2) develop a decision-adjusted modeling framework to predict high risk drivers based on telematics data. The decision-adjusted framework optimizes predictive performance based on the objective of the study, e.g., top 1% of high risk drivers. In a case study, we developed an optimal driver level risk prediction model based on the telematics data (high G-force events) and driver demographic information using the SHRP2 NDS.
This webinar features research from Safe-D Project 01-001: Big Data Methods for Simplifying Traffic Safety Analyses