The momentum of transformative technologies in transportation is unprecedented. Stories on advanced vehicles and infrastructure appear every day in popular media, highlighting our selected disruptive themes and applications. Yet, these disruptors will need considerable development over the next few decades, creating a high workforce demand. Transportation companies are recruiting from universities in increasing numbers (e.g., General Motors recently held a recruiting event at VTTI with over 300 hundred students), and new transportation job opportunities are abundant (e.g., Google has 36 jobs currently advertised for their self-driving car program). The Safe-D National UTC views this new demand as both an opportunity and a responsibility to attract and prepare the next generation of transportation researchers and workers.
To build upon this existing interest and ensure that students see transportation as we do (as an exciting area for the application of next-generation and life-saving technologies), it is critical to connect with future transportation leaders at all stages of education and career development. Accordingly, Safe-D is committed to prioritizing education and workforce development by leveraging existing university programs and creating entirely new projects and programs using UTC support.
In addition to requiring all Safe-D research projects to include significant student involvement, the Safe-D National UTC has set-aside funding for student support through various projects and programs. A description of the student projects supported by Safe-D is below.
Safe Control Technologies for Autonomous Vehicle Platoons (TTI/TAMU)
Led by Mike Lukuc (TTI/TAMU)* and Andy Hwang (TAMU), this project supported a student in support of TxDOT project 0-6836 Commercial Truck Platooning. Under this project, the student funded by Safe-D is responsible for knowledge transfer of the current control algorithm from the vendor, coding that algorithm into new model-based software architecture, and simulation-based software verification for the baseline and future algorithm iterations. This is a key component of an R&D path to utilize a simulation-based software development approach that improves efficiency by reducing time, complexity, risks, uncertainty, and costs associated with testing highly dynamic, failure mode, and crash-imminent platooning scenarios.
The report from TxDOT Project 0-6836: Commercial Truck Platooning—Level 2 Automation is available here.
Development of Analytic Method to Determine Weaving Patterns for Safety Analysis near Freeway Interchanges with Access Management Treatments (TTI/TAMU)
This project supports a student in support of NCHRP Project 07-23 Access Management in the Vicinity of Interchanges and was led by Karen Dixon (TTI/TAMU)* and Maryam Shirinzadeh Dastgiri (TAMU). This project used a large volume of operational field data, micro-simulation data, and crash data to identify linkages between interchange design, driveway placement, and upstream major intersection locations that affect corridor safety. The effort primarily focused on the diamond interchange configuration that is very common in the United States. The methods for determining corridor safety near interchanges can create challenges. Due to the large traffic volumes, varying travel times, widely varying geometric features of the interchange, and a variety of access management scenarios, it is a challenge to assess the data and determine if safety performance issues should be attributed to the interchange traffic, the driveway traffic, the upstream intersection traffic, or a combination of these operational features blended with the prevailing corridor geometrics. These unknown issues make improving safety at these locations a challenge. For this effort, the student used field-collected travel time and volume information to develop validated micro-simulation models that depict actual site conditions. Each location will then undergo a safety assessment to identify what factors appear to be correlated with safety performance. These “base” models will then be modified to change access management techniques (add or change medians, increase or decrease access density, shorten or lengthen distance to nearest major intersection) and then assess how minor changes may influence the operational performance. Though safety cannot be directly extracted from this micro-simulation activity, performance measures attributed to safety for the “base” models will be identified and evaluated using sensitivity analysis for the resulting large volume of data. The student funded by Safe-D is working with data collected for NCHRP project 07-23 but she is providing an added value by developing a method for determining vehicle weaving patterns in these interchange regions. This activity goes beyond the scope of the NCHRP project but builds upon it. The student’s work requires data mining using simulation data, video data, and travel time data (in some cases all merged together).
Developing Systems for Autonomous Vehicles to Identify and Localize Emergency Vehicles (TTI/TAMU)
Within the CAST (Connected Autonomous Safe Transportation) Program, one of the projects is an exploration to determine how an autonomous vehicle should respond to emergency vehicles on the road. There are three threads of investigation:
- Determination of the “right” or “ideal” response. We are interacting with the TEEX Law Enforcement and Security Training, and through them with local police and fire department personnel, to identify key traffic scenarios involving emergency vehicles, and the possible sets of good and bad responses from an autonomous vehicle.
- Identification and possible Localization of an Emergency Vehicle. This would be a combination of detection and identification of sound and visual signals from an Emergency Vehicle. We will primarily be evaluating different AI/Machine Learning techniques.
- Response execution based on the identification of an Emergency Vehicle. We already have an autonomous vehicle within CAST that can be programmed as desired. We will be implementing and demonstrating the desired emergency response on this vehicle.
During summer 2017, TTI/TAMU faculty (Sivakumar Rathinam (TAMU)*, Swaminathan Gopalswamy (TTI/TAMU), Geethik Narayana Kamineni, Zhiyong Yang, Sheelabhadra Dey) led students assigned to this project to complete the following tasks:
- Investigate the use of various machine-learning techniques to process sound data, and identify emergency vehicles. The sound signals were processed to extract several key sound features, which were then used as the basis for performing the identification of the type of the sound signal. Learning models were trained offline using a database of different sound signals to perform the identification. Then these training models were adapted for real-time application to sound signals captured directly from microphones. The performance of these identification algorithms was verified using real microphones listening to recorded emergency vehicle sounds.
- Begin experimentations, in collaboration with TEEX Law Enforcement and Security Training, to recreate typical traffic scenarios of interest in the context of emergency vehicles. Specifically, we performed experiments where an autonomous vehicle was instrumented with multiple microphones and cameras, and two emergency vehicles made simulated runs with sirens on and emergency lights flashing. This data has been collected and is being processed.
The end-goal of the summer work was to lead to a formal proposal for sustained research into this important safety consideration for autonomous vehicles, namely, how should autonomous vehicles respond to emergency vehicles on the road. This project was ultimately funded by Safe-D (Safe-D Project 03-051: Response of Autonomous Vehicles to Emergency Response Vehicles). The project also resulted in a conference paper: Nayak, A. S. Dey, S. Rathinam, S. Gopalswamy,3rd Annual Texas A&M Transportation Technology Conference, May 2018 (Published).
Motorcycle Crash Data Analysis to Support Implementation of a Concrete Barrier Containment Options for Errant Motorcycle Riders (TTI/TAMU)
TTI/TAMU researchers Chiara Silvestri-Dobrovolny (TTI/TAMU)*, Heather Sulaica (TAMU), and Johnathan Luke Wilson (TAMU) led this student project during the summer of 2017. The objectives of this project were to review and analyze existing crash data on motorcycle-related accidents, as well as to conduct a detailed literature review on existing motorcycle testing standards and various protocols that foreign Countries have developed throughout the years. The students involved in this project have assisted with crash data review from the Texas CRIS database. They have analyzed motorcycle crash data that was recorded in the State of Texas and that occurred specifically on a Flyover-Connector road type or at a curve. In addition, the students reviewed available police narratives documents from the crashes which occurred on Flyovers or Connectors, to determine, when possible, the dynamic of the accident and to verify other detailed information that was not necessarily present in the crash data file. The students also utilized available computer programs to obtain pictures of those locations where the accidents occurred and complement missing information from the narratives. Students also performed a detailed international literature review of existing standards and protocols on motorcycle testing against roadside safety barriers, as well as of current practices of retrofitting existing roadside safety systems to enhance motorcycle rider safety. This UTC project provides the ability to perform detailed research and data investigation complementing an existing TxDOT project (490027-3 – internal #). The funded TxDOT project aimed at exploring design options for a retrofit concrete barrier system to be deployed at appropriate bridge locations to address errant motorcycle riders’ safety. The objective of this project includes the design, development, and evaluation through computer simulations of an improved retrofit barrier system(s) that has the capability to safely contain errant motorcycle riders during an impact event. As the project evolved, it was ultimately supported as a full research project (Safe-D Project TTI-Student-04), and is expected to generate a full report (expected to be finalized in Summer 2019).
PREPARES Student Support (VT)
In the Fall of 2018, a team of VT graduate students in Safe-D Director Zac Doerzaph’s Advanced Vehicle Safety Systems (BMES 5984) class were tasked with the challenge of developing a novel safety system to address a motor vehicle safety issue. This academic challenge produced the Pre Rear-End Positioning and Risk Extenuation System (PREPARES), a new system aimed at protecting the occupants of a struck vehicle in the case of an unavoidable rear-end collision. After the completion of the class project, the team decided that the system could be further developed and entered into an international competition through NHTSA. The Safe-D National UTC committed to supporting the students in their continued development of the system through federal and matching funding sources. PREPARES rapidly redirects the occupant’s attention in a manner that elicits optimal seating position in the milliseconds prior to impact, mitigating the effects of whiplash and other soft tissue injuries. The system relies on a compact array of sensors to reliably measure the position and velocity of vehicles approaching from behind. An algorithm pre-processes the raw sensor data, determines if the approaching vehicle is a collision threat, and issues a warning by comparing measured distance and velocity to the predicted stopping distance. The warning interface consists of salient audible and visual components. The goal is to create a near-automatic human response that repositions the occupant’s torso and head into position for impact with the seat and headrest, based on eliciting a strong desire to quickly attend to the alert.
On April 19, 2019, the PREPARES Student Team was also selected as a North American Region Winner for the Student Safety Technology Design Competition (SSTDC) of the Enhanced Safety of Vehicles (ESV) 26th International Technical Conference. The team will compete in the final, international competition in Eindhoven, Netherlands in June 2019.
The team also submitted a report to NHTSA for the SSTDC, which can be found here: PREPARES Report (pdf)
Exploring Crowdsourced Monitoring Data for Safety (TTI)
This project encompasses four different activities to explore safety applications of emerging crowd-sourced data and datasets available from commercial aggregators. The first activity examines systems used to monitor and count pedestrian activity. Developing crash rates for these vulnerable users depends on knowing the volume of activity. Data from metropolitan planning organizations as well as commercial and community-based reports of bicycle and pedestrian activity will be assessed for accuracy by comparing them to manual counts. The second activity will examine waypoint travel pattern data from a commercial data aggregator to determine its applicability to safety analyses. The evaluation will assess the geographic and temporal distribution and representativeness of the sample as well as how roadways of different functional classes are represented. The third activity will examine data from a crowd-sourced traveler information app to determine if incident reports in the app correspond to official incident records. The task will develop a data catalog identifying data attributes, coverage areas, resolution of the data, and timeliness of the data. Overall these three exploratory efforts will provide future research projects an understanding of what is available from these new big data sources and how they could be used to improve safety. As the project evolved, it was ultimately supported as a full research project (Safe-D Project TTI-Student-05), and is expected to generate a full report (expected to be finalized in Fall 2019).
Quantifying the Benefits and Harms of Connected and Automated Vehicle Technologies to Public Health and Equity (TTI)
Automated vehicle technologies (AV) have the potential to become one of the most highly disruptive technological applications of our century. AV technologies represent a switch in driving responsibility from human to machine. They encompass a diverse range of automated technologies, from relatively simple driver assistance systems to fully automated, driverless
vehicles. Because 94% of crashes are attributed to human error, the safety benefits that AVs could provide are compelling—although incontrovertible empirical proof that AVs deliver safety benefits has yet to be produced. Other anticipated benefits of AVs are related to the potential mitigation of congestion, air pollution, greenhouse gases (GHG), and mobility enhancement for underserved populations, which could impact public health significantly. Because they represent a disruptive innovation, AVs have attracted attention from various areas of research such as driver behavior, land use, roadway design, transport policy, etc. However, there has been less focus on the public health impacts of AVs. The implications of AVs on health are very complex, and modeling them requires an interdisciplinary effort. This study is an attempt to quantify the AVs’ impacts on public health and health equity through the changes in transportation. To this end, we focused on two risk factors, motor vehicle crashes, and air pollution.
Detecting Pavement Distresses Using Crowdsourced Dashcam Camera Images (TTI)
Pavements play a vital role in the transportation infrastructure in the United States. Damage to public road transportation infrastructure causes roadways to fail to perform as intended and increases crash risks. Road damage must be detected quickly and accurately in order to maintain roads and effectively allocate repair money. In this study, four deep-learning objects detection models were used for detecting five types of pavement damage using Nexar Dashcam images. The single-shot multi-box detector (SSD) and faster region-based convolutional neural networks (Faster R-CNN) object detection models using MobileNet and Inception techniques were applied to the selected and processed images. An attempt was made to detect alligator cracking, longitudinal cracking, transverse cracking, and patching on the roadway surface using the developed model. A total number of 6,326 images with a total number of 8,970 damage instances were selected from the provided data. Faster R -CNN models showed higher recall and precision compared to the SSD models. Faster R-CNN Inception Resnet showed the highest average precision of 56%, followed by Faster R-CNN Inception with an average precision of 50%. Recall values showed almost the same trend as precision. Faster R-CNN showed the highest average recall of 43%. The results showed that Type-I and Type-II damages were detected with the highest recall and precision compared to other damages. Type-I damage was detected with an average precision of 81% and recall of 70 % in all of the models.
Assessment of Work Zone Pre-crash Scenarios Using Crowdsourced Data (TTI)
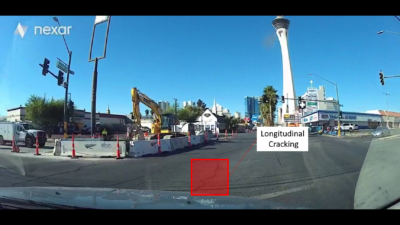
Work zones are one of the hot spots with an increased risk of crashes. Work zones require that drivers temporarily change their travel path and travel lanes, which can increase the crash probability if the drivers are engaged in distracted behaviors and are not able to see the warning signals and pavement markings. In recent years, the diffusion of emerging technologies facilitated collecting high-quality data from vehicles that provide safety researchers with the opportunity to explore the sequence of events leading to crashes, as driver behavior. Identifying dangerous scenarios to prevent their occurrence is a problem at the very core of intelligent transportation systems (ITS) research. Nowadays, vehicles can easily be equipped with not only the standard telematics sensors, such as accelerometers and gyroscopes but also video cameras such as dashcams that can help to detect unsafe situations that lead to traffic crashes. The problem of the crash and near-crash event identification calls for the detection and classification of driving events using vehicle data collected from onboard devices. The objective of this study is to explore the potential of dashcam data for conducting pre-crash scenario analysis for work zones. In this project, the research team will identify unsafe driving events at work zones from videos collected by Nexar’s front-facing dashboard camera (dashcam) and trajectory data. The results of this project could be used for developing automated driving systems (ADS) and automated driver assistance systems (ADAS) to improve safety in work zones.
Identifying Deviations from Normal Driving Behavior (TTI)
Advanced driver assistance systems (ADAS) have significantly improved safety on today’s roadways but their impact may be limited by driver errors. Understanding and identifying these driver errors will require the integration of multi-domain datasets through predictive modeling and data integration approaches. The goals of this project are to identify relevant datasets for ADAS error prediction, evaluate modeling approaches for predicting driver errors during ADAS use, and develop models to proactively predict driver errors. Results from the project will be used to guide data collection system design at automakers and develop predictive modeling benchmarks.
Evaluating Emotion Regulation Techniques for Supporting Driving Safety and Performance (TTI)
Humans operating motor vehicles are often required to engage in decision-making while under substantial cognitive loads imposed by the driving environment itself. Experiencing elevated emotions can influence driver decision-making in a way that increases the risk to the safety of the driver and system performance. Emotion regulation techniques (ERTs) can be used to mprove driving safety and performance; however, these techniques can only be effective in an operational driving context if they impose minimal additional cognitive loading on the driver, thus avoiding issues with distraction and the increased workload that could further impact decision making, driving performance, and safety. Additionally, due to motivational factors influencing individual drivers, ERTs that can be activated with greater subtlety (less obvious to the driver) may be more effective than those that are perceived as more obvious and potentially condescending. To determine effective methods that ultimately improve driving safety, two classes of ERTs will be evaluated in this study: those that are classified as “overt”, such as explicitly prompting drivers to perform a cognitive reappraisal of the situation, and those classified as “covert”, such as introducing subtle cues that influence physiological systems, such as synchronizing breathing patterns in a manner that is effective in regulating emotions. Given that affective states can be manipulated with little or no conscious engagement, covert ERTs that minimize cognitive demand, and perhaps even work subconsciously, may be well suited for supporting drivers in an active driving context. The findings of this work can provide design guidance for future driver systems design.
Deep Learning Video Feature Representation Models for Driving Scenario Assessment (VTTI/VT)
VTTI researcher Feng Guo led the supported VT student Liang Shi for the summer of 2022. This study developed a novel AI model to detect crash based on front videos collected from naturalistic driving study data. Accurate crash detection is crucial in emergency response, active safety systems development, navigation, and automated driving. The advancement in camera systems, computer vision, artificial intelligence, and computing power has made crash detection a viable and efficient approach. However, traditional time series models like Long short-term memory (LSTM) and Convolutional neural network (CNN) face challenges in detecting crash accurately from video clips. To address these limitations, we proposed a novel two-stream crash detection method that combines optical flow and deep learning with a focus on spatial-temporal modeling and multi-type event prediction. Our research makes two main contributions to the field: 1) the two-stream network improves crash detection performance by combining optical flow and TimeSFormer, and 2) multi-head attention on optical flow frames for end-to-end crash detection. Our approach surpasses the limitations of traditional CNN+LSTM model and enhances the extraction of information critical for collision detection.