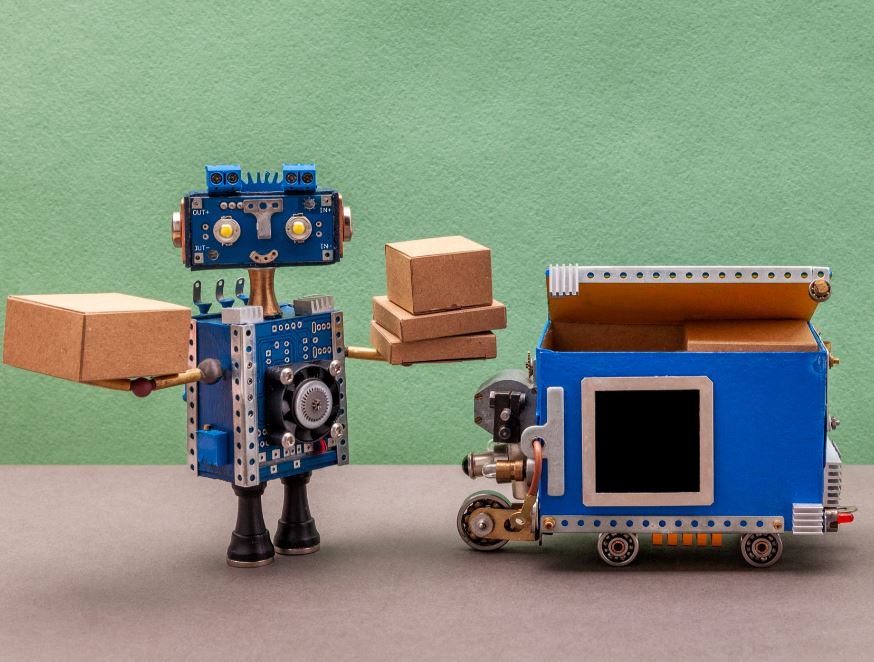
Abstract
The National Highway Traffic Safety Administration (NHTSA) recently granted permission to deploy low-speed autonomous delivery vehicles (ADVs) on roadways. Although the mobility of ADVs is limited to low-speed roads and these vehicles are occupant-less, frequent stops and mobility among residential neighborhoods cause safety-related concerns. There is a need for a comprehensive safety impact analysis of ADVs. This study examined the safety implications and safety impacts of ADVs by using novel approaches.
The objective of this study is to understand the safety-related issues associated with ADVs. Due to the limitation of acquiring large-scale vehicle movement data from ADV operators, this study used alternative datasets to achieve the research objectives. This study performed two levels of safety analysis: 1) safety implication analysis using datasets that imply the scenarios of ADVs, and 2) safety impact analysis using datasets from real-world ADVs. To perform the safety implication and safety impact analysis, the research team selected the following datasets: 1) Fatality Analysis Reporting System (FARS) [2016-2020], 2) Crash Report Sampling System (CRSS) [2016-2020], 3) California AV and ADV collision Data [2014-2020], 4) Waymo Open Data, and 5) Third-party ADV Trajectory Data (sample data).
Association rules mining was applied to the datasets to identify significant patterns. This study generated a total of 80 association rules which provide risk patterns associated with ADVs. The rules can be used as prospective benchmarks to examine how these rule-based risk patterns can be reduced by ADVs that replace human-driven trips.
Project Highlights
- This study used five different datasets to explore the safety-related issues associated with ADVs.
- This study generated a total of 80 association rules (in the form of risk patterns) that provide risk patterns associated with ADVs. The rules can be used as prospective benchmarks to examine how these rule-based risk patterns can be reduced by ADVs that replace human-driven trips.
- The Safe System-based ADV-related decision support tool can be used for infrastructure readiness for the ADVs.
Final Report
EWD & T2 Products
Undergraduate and graduate courses:
- TTI/Texas A&M/University of San Antonio (UTSA)/Texas State University (TXST). Course materials are available on the GitHub repository of Dr. Subasish Das. Some of the material will be included in the slides and class notes for the following graduate courses:
- CVEN 626 (Highway Safety) at TAMU
- CE5493: Traffic Engineering at UTSA
- CE 4361: Highway Engineering at Texas State University.
Student Impact Statement(pdf): Two students received funding under this project (Zihang Wei, a Ph.D. Civil and Environmental Engineering student from Texas A&M, and Valerie Vierkant, an Undergraduate Psychology student from Texas A&M University). This file contains examples of how this project had an impact on their education and workforce development.
Decision Support Tool – The developed decision support tool matrix based on Safe System. This file comes directly from the final report.
Presentations/Publications
Das, S. (2021, November 24). New Frontiers in Highway Safety Research: Disruptive Technologies and Automated Vehicles. UL Lafayette Civil Engineering Department Invited Talk. [Virtual Webinar]
Das, S. (2022, October 22). Autonomous Delivery Vehicle as a Disruptive Technology: How to Shape the Future with a Focus on Safety? [SafeD Virtual Webinar available here.
Das, S., (2022). Use of Safe System Approach for Automated Vehicles. Transportation Research Record: Journal of the Transportation Research Board (under review).
Das, S., and Kutela, B. (2022). Using Advanced Language Models to Understand News Media Coverage of Automated Delivery Vehicles. Transportation Research Record: Journal of the Transportation Research Board (under review).
Das, S. (2022). Artificial Intelligence in Highway Safety. CRC Press, Boca Raton, FL.
Final Dataset
The final datasets for this project are located in the Safe-D Collection on the VTTI Dataverse; DOI: 10.15787/VTT1/VAEBNM.
Research Investigators (PI*)
Subasish Das (TTI/TAMU)*
Ioannis Tsapakis (TTI/TAMU)*
Zachary Elgart (TTI/TAMU)
Boniphace Kutela (TTI/TAMU)
Eric Li (VT/VTTI)
Zihang Wei (Student-TTI/TAMU)
Ly-Na Tran (Student-TTI/TAMU)
Project Information
Start Date: 2020-01-10
End Date: 2022-05-31
Status: Complete
Grant Number: 69A3551747115
Total Funding: $803,700
Source Organization: Safe-D National UTC
Project Number: 05-087
Safe-D Theme Areas
Safe-D Application Areas
Risk Assessment
Planning for Safety
Performance Measures
Sponsor Organization
Office of the Assistant Secretary for Research and Technology
University Transportation Centers Program
Department of Transportation
Washington, DC 20590 United States
Performing Organization
Texas A&M University
Texas A&M Transportation Institute
3135 TAMU
College Station, Texas 77843-3135
USA
Virginia Polytechnic Institute and State University
Virginia Tech Transportation Institute
3500 Transportation Research Plaza
Blacksburg, Virginia 24061
USA