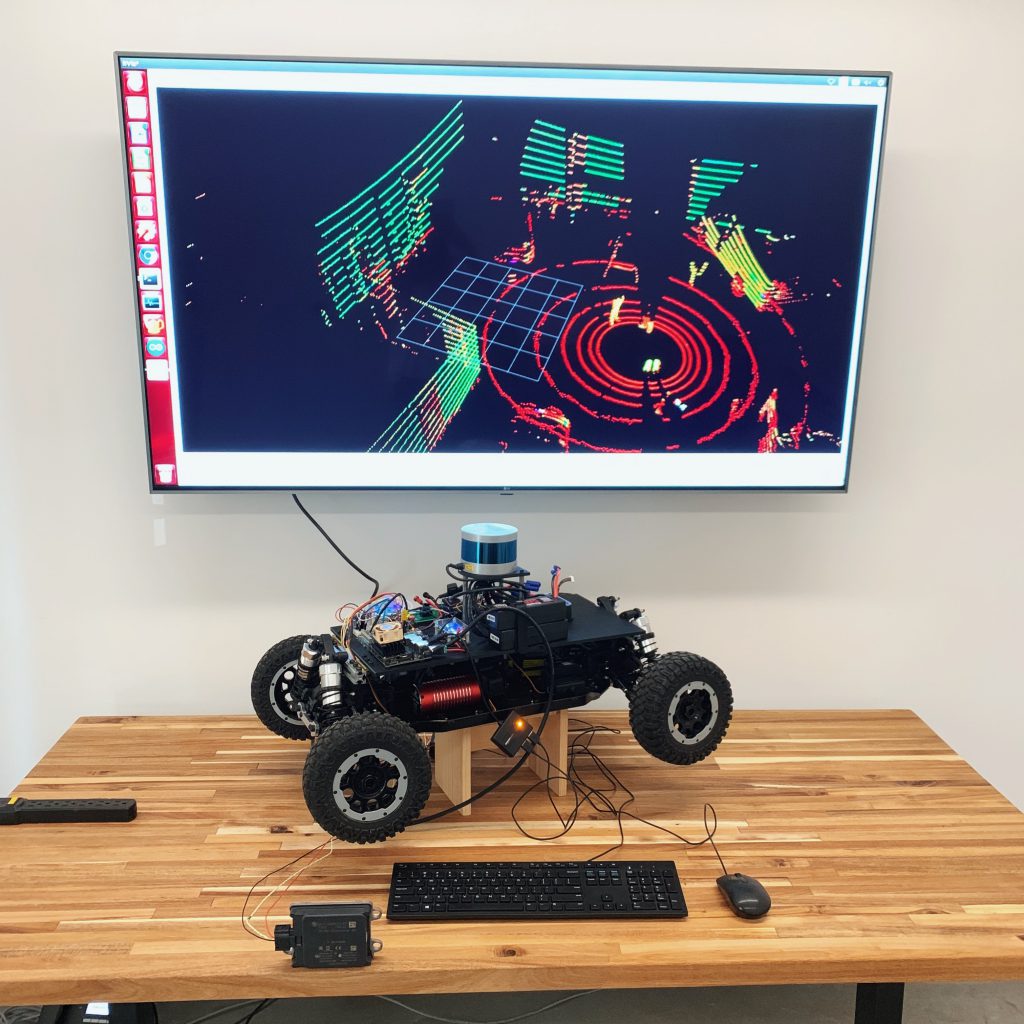
Abstract
R&D platforms and robust prototypes are essential for the development and demonstration of automated driving functions. Thousands of hours of safety and performance benchmarks must be met before an ADAS system is production-ready. However, full-scale test-beds are expensive to make, labor-intensive to design, and include inherent safety risks when testing. To reduce these risks and expenses, scaled prototypes can be developed to model system design and vehicle behavior in targeted driving scenarios. Scaled test-beds can improve the ease of safety-testing future ADAS systems and can help visualize test results and system limitations to audiences with varying technical backgrounds better than software simulations. However, though small-scaled vehicles can accommodate similar on-board systems to its full-scale counterparts, the performance of these systems (particularly sensors) can be affected. For example, as the vehicle scales down, the resolution from perception sensors and the performance for object tracking decreases, especially from mounted radars. With many automated driving functions relying on radar tracking, the small-scaled vehicle must overcome the reduced tracking ability at scale in order to provide safe and accurate system modeling. This project proposes a sensor fusion approach to augment radar data in a scaled environment that uses an off-the-shelf LiDAR as a high spatial resolution sensor. It is expected that, with this approach, radar tracking software (RTS) developed at full-scale will also reliably operate in small-scale environments. Successfully implementing radar and LiDAR fusion, system tests with scaled test-bed vehicles can then identify safety concerns in ADAS functions more quickly and efficiently, leading to faster and safer product development.
Project Highlights
- The aim of this work was to establish a method of including radar sensor tracking capabilities in small-scale testbed vehicles. The team researched and implemented a high-level sensor fusion approach between radar and LiDAR to address several questions regarding this topic.
- While LiDAR improved the small-scale testbed tracking performance, it did not reach the level of tracking performance observed for the full-scale testbed. Thus, the track fuser improved upon but did not totally restore, the tracking accuracy of the full-scale radar tracks. Additional performance enhancements, however, are possible.
- This investigation demonstrated the ability to use radar in small-scale environments with an associated track fuser, particularly during offline analysis of sensor recordings. The simplified LiDAR clustering and Kalman Filter implementations make the approach accessible to many small-scale testbed architectures that already implement a LiDAR sensor.
- The project supported one graduate student and two undergraduates under the VTTI InternHUB program umbrella.
Final Report
Final Report Safe-D VTTI-00-025
EWD & T2 Products
A set of lecture notes related to the project was developed for a graduate-level class centered on ADAS
Student thesis: Beale, G.T. (2021). Radar and LiDAR fusion for scaled vehicle sensing. Unpublished master’s thesis. Virginia Tech.
BMES 5984 – Advanced Vehicle Safety used materials that were developed from this project. These course materials can be downloaded here – (Course Slide Deck and Course Notes for each subject)
Student Impact Statement – Gregory Beale (pdf): The student(s) working on this project provided an impact statement describing what the project allowed them to learn/do/practice and how it benefited their education.
This project provided two exhibits centered on radar and LiDar systems in autonomous vehicles at the Virginia Tech Science Festival (over 6,000 attendees) and local elementary school STEM night (several dozen attendees).
Presentations/Publications
A publication, in the form of a journal article that summarizes the results of the project, is currently being developed.
Theory and configuration of the system behind the LiDAR and radar fusion (described in detail within the aforementioned final report and master’s thesis)
Final Dataset
The final datasets for this project are located in the Safe-D Collection on the VTTI Dataverse; DOI:10.15787/VTT1/BQJRFN.
Research Investigators (PI*)
Miguel Perez (VTTI)*
Zac Doerzaph (VTTI/VT)
Greg Beale (VT-Student)
James H. Critchley (VT-Student)
Sean Copenhaver (VT-Student)
Alexander Broz (VT-Student)
Project Information
Start Date: 2019-11-25
End Date: 2021-06-30
Status: Closed
Grant Number: 69A3551747115
Total Funding: $142,049.00
Source Organization: Safe-D National UTC
Project Number: VTTI-00-025
Safe-D Theme Areas
Automated Vehicles
Transportation as a Service
Safe-D Application Areas
Vehicle Technology
Risk Assessment
Performance Measures
More Information
Sponsor Organization
Office of the Assistant Secretary for Research and Technology
University Transportation Centers Program
Department of Transportation
Washington, DC 20590 United States
Performing Organization
Virginia Polytechnic Institute and State University
Virginia Tech Transportation Institute
3500 Transportation Research Plaza
Blacksburg, Virginia 24061
USA