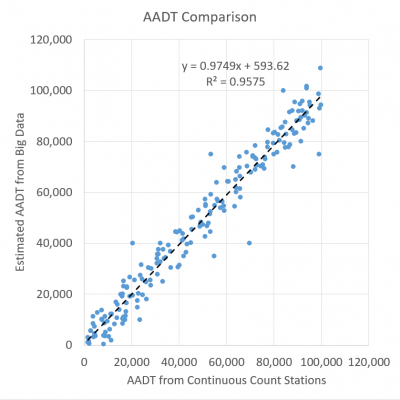
Abstract
The goal of this project is to examine whether traffic volume estimates developed from disruptive technologies such as cell phones, GPS/Bluetooth devices, and alternative data sources (e.g., demographic, socioeconomic, land use data) can be used confidently and accurately to support data-driven safety analysis (i.e., network screening) to meet the 2016 Highway Safety Improvement Program (HSIP) Final Rule requirements. The main research questions include: What is the expected accuracy of AADT estimates developed from disruptive technologies? How does the AADT accuracy vary by roadway functional class for urban and rural roads? What is the average penetration rate of disruptive data sources? What is the impact of underestimating or overestimating AADT on data-driven safety analysis? This research involves conducting a review of the literature; gathering and integrating several datasets from Texas and Virginia such as crash, roadway, and traffic data, including Annual Average Daily Traffic (AADT) estimates developed from disruptive data sources; performing statistical analysis and validating AADT estimates from disruptive technologies, and conducting an impact analysis to determine how AADT estimation errors can affect the results of safety analysis.
Project Highlights
- The comparison between DOT and SLD AADT estimates revealed that the median APE for roads with AADT greater than 2000 vpd is approximately 25%.
- The AADT accuracy gradually improves from lower to higher traffic volume roads.
- SLD tends to overestimate AADT within lower volume ranges (0-10,000 vpd) and underestimate it for roads that have an AADT higher than 10,000 vpd.
- Decision trees can improve the performance of traditional SPFs.
- The model form is of high importance in SPF development. Negative binomial Lindley (NB-L) models provided a better fit compared to other traditional models.
- The dispersion structure is highly dependent upon the underlying negative binomial formulation. Different variance structures did not considerably change the model performance; however, in highly skewed datasets, flexible variance structures can provide more flexibility to the model.
Final Report
EWD & T2 Products
TTI/Texas A&M: CVEN 626 – Highway Safety (Fall 2021): Some of the material will be included in the slides and class notes for the graduate course CVEN 626. At the time this report was written, the class notes had not been yet updated. (Slides Coming Soon)
TTI/Texas A&M: Some of the material has been included in Chapter 2 of the forthcoming textbook titled “Highway Safety Analytics and Modeling” co-written by Dr. Dominique Lord which will be published on March 1, 2021.
Student Funding and Enrichment:
TTI – one Ph.D. student, Ali Khodadadi, at the Texas A&M University. Title of the dissertation to be determined. Status: anticipated December 2021.
TTI – one undergraduate student, Jessica Morris, at the University of Texas-San Antonio.
Student Impact Statement – Ali Khodadadi & Jessica Morris (pdf): The student(s) working on this project provided an impact statement describing what the project allowed them to learn/do/practice and how it benefited their education.
New SPFs for NFAS roads that TxDOT and VDOT can use in the data-driven safety analysis.
Presentations/Publications
Das, S., I. Tsapakis, and A. Khodadadi. Safety performance functions for low-volume rural minor collector two-lane roadways. IATSS Research, 2021. https://doi.org/10.1016/j.iatssr.2021.02.004
Khodadadi, A., I. Tsapakis, S. Das, D. Lord and Y. Li. Application of Different Negative Binomial Parameterizations to Develop Safety Performance Functions for Non-Federal Aid System Roads, Accident Analysis and Prevention, 156, June 2021. https://doi.org/10.1016/j.aap.2021.106103
Khodadadi, A., I. Tsapakis, S. Das, D. Lord and Y. Li. Application of Different Negative Binomial Parameterizations to Develop Safety Performance Functions for Non-Federal Aid System Roads. Presented at the 100th Annual Meeting of the Transportation Research Board, January 2021.
Das, S., I. Tsapakis, and A. Khodadadi. (2021). Use of Disruptive Technologies to Support Safety Analysis and Meet New Federal Requirements. US Department of Transportation SAFE-D National Transportation Center Webinar Series, March 25, 2021.
Final Dataset
The final datasets for this project are located in the Safe-D Collection on the VTTI Dataverse; DOI: 10.15787/VTT1/HMQZOE.
Research Investigators (PI*)
Ioannis Tsapakis (TTI/TAMU)*
Subashish Das (TTI/TAMU)
Eric Li (VTTI)
Dominique Lord (TAMU)
Alfredo Sanchez (TTI)
Lorenzo Cornezo (TTI)
Project Information
Start Date: 2019-08-01
End Date: 2021-07-21
Status: Active
Grant Number: 69A3551747115
Total Funding: $1,607,000
Source Organization: Safe-D National UTC
Project Number: 04-113
Safe-D Theme Areas
Safe-D Application Areas
Planning for Safety
Freight and Heavy Vehicles
Performance Measures
More Information
Sponsor Organization
Office of the Assistant Secretary for Research and Technology
University Transportation Centers Program
Department of Transportation
Washington, DC 20590 United States
Performing Organization
Texas A&M University
Texas A&M Transportation Institute
3135 TAMU
College Station, Texas 77843-3135
USA
Virginia Polytechnic Institute and State University
Virginia Tech Transportation Institute
3500 Transportation Research Plaza
Blacksburg, Virginia 24061
USA