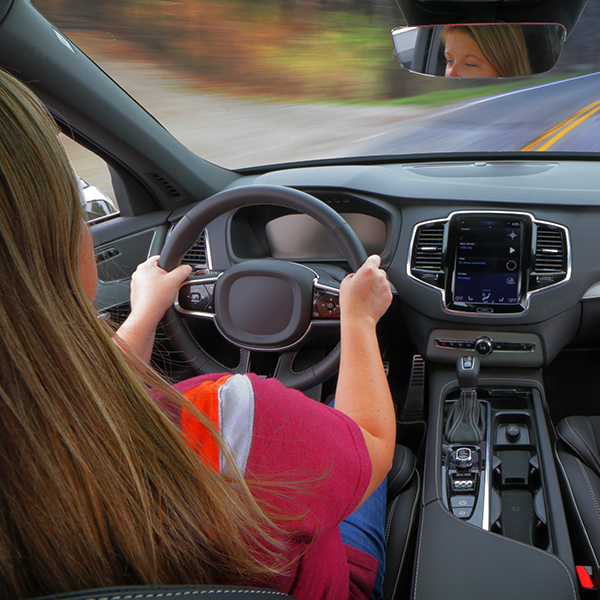
Abstract
This report gives an overview of the main findings from the Behavior-based Predictive Safety Analytics – Pilot Study project. The main objective of the project was to investigate the possibilities of developing statistical models predicting individual driver crash involvement based on individual driving style, demographic and behavioral history variables, using large sets of naturalistic driving data. The project was designed as a pilot project with the objective to provide the basis for a future more comprehensive research effort. Based on the SHRP2 data, a subset of behavior and crash data including 2458 drivers was created for the present analysis. The data was analyzed to investigate to what extent these drivers were differentially involved in crashes and near crashes, to what extent this was associated with individual characteristics and if it is possible to predict individual drivers’ crash and near crash involvement based on variables representing individual characteristics. The results clearly demonstrated the presence of differential crash and near crash involvement and showed significant associations between enduring personal factors and crash involvement. Moreover, logistic regression and random forest classifiers were relatively successful in predicting crash and near crash involvement based on individual characteristics while the ability to specifically predict involvement in crashes was more limited.
Project Highlights
Crashes generally occur due to interactions between enduring and temporal personal factors and situational factors, and can thus potentially be predicted, at least to some extent, using variables that reflect individual characteristics.
Driver Behavior Questionnaire violations factor and sensation seeking scores showed moderate correlations (around 0.2) with near-crashes, certain driving style measures (e.g., hard turns) and age, but low (0.02-0.1) correlations with crashes, depending on crash severity and at fault classification.
The behavior observation data should include driving exposure data in mileage and/or driving hours and preferably be recorded continuously in order to obtain true rates of behavioral events.
Commercial naturalistic datasets contain larger numbers of crashes but are, due to their event-based nature, typically limited with respect to the information available on behavior in non-conflict situations.
Final Report
02-020 Final Research Report (PDF)
EWD & T2 Products
Project Summary (pptx): This file contains a slide deck summarizing the results from this project’s investigation into opportunities and barriers for utilizing commercial naturalistic crash data in academic research.
Student Impact Statement (pdf): One student was funded under this project (Wenyan Huang from VT, Ph.D. candidate). This file contains a statement of the impact this project made on this student’s education and workforce development.
Presentations/Publications
Huang, W., Engstrom, J., Miller, A., Dreger, F. A., Soccolich, S., de Winter, J. C. F., & Ghanipoor Machiani, S. (2018). Analysis of Differential Crash and Near-Crash Involvement Based on Naturalistic Driving Data. Presented at the 7th International Symposium on Naturalistic Driving Research. Blacksburg, Virginia. (Accepted)
De Winter, J. C. F, Dreger, F. A., Huang, W., Miller, A., Soccolich, S., Ghanipoor Machiani, S., & Engstrom, J. (2018). The relationship between the Driver Behavior Questionnaire, Sensation Seeking Scale, and recorded crashes: A brief comment on Martinussen et al. (2017) and new data from SHRP2. Accident Analysis and Prevention, 118, 54-56. (Accepted)
Final Dataset
The final datasets for this project are located in the Safe-D Collection on the VTTI Dataverse; DOI: 10.15787/VTT1/464GB9.
Research Investigators (PI*)
Andrew Miller (VTTI)*
Sahar Ghanipoor Machiani (SDSU)
Project Information
Start Date: 2017-05-01
End Date: 2018-7-31
Status: Complete
Grant Number: 69A3551747115
Total Funding: $ $72,623
Source Organization: Safe-D National UTC
Project Number: 02-020
Safe-D Theme Areas
Safe-D Application Areas
Risk Assessment
Driver Factors and Interfaces
Vehicle Technology
Freight and Heavy Vehicle
More Information
RiP URL
UTC Project Information Form
Sponsor Organization
Office of the Assistant Secretary for Research and Technology
University Transportation Centers Program
Department of Transportation
Washington, DC 20590 United States
Performing Organization
Virginia Tech Transportation Institute
3500 Transportation Research Plaza
Blacksburg, Virginia 24061
USA