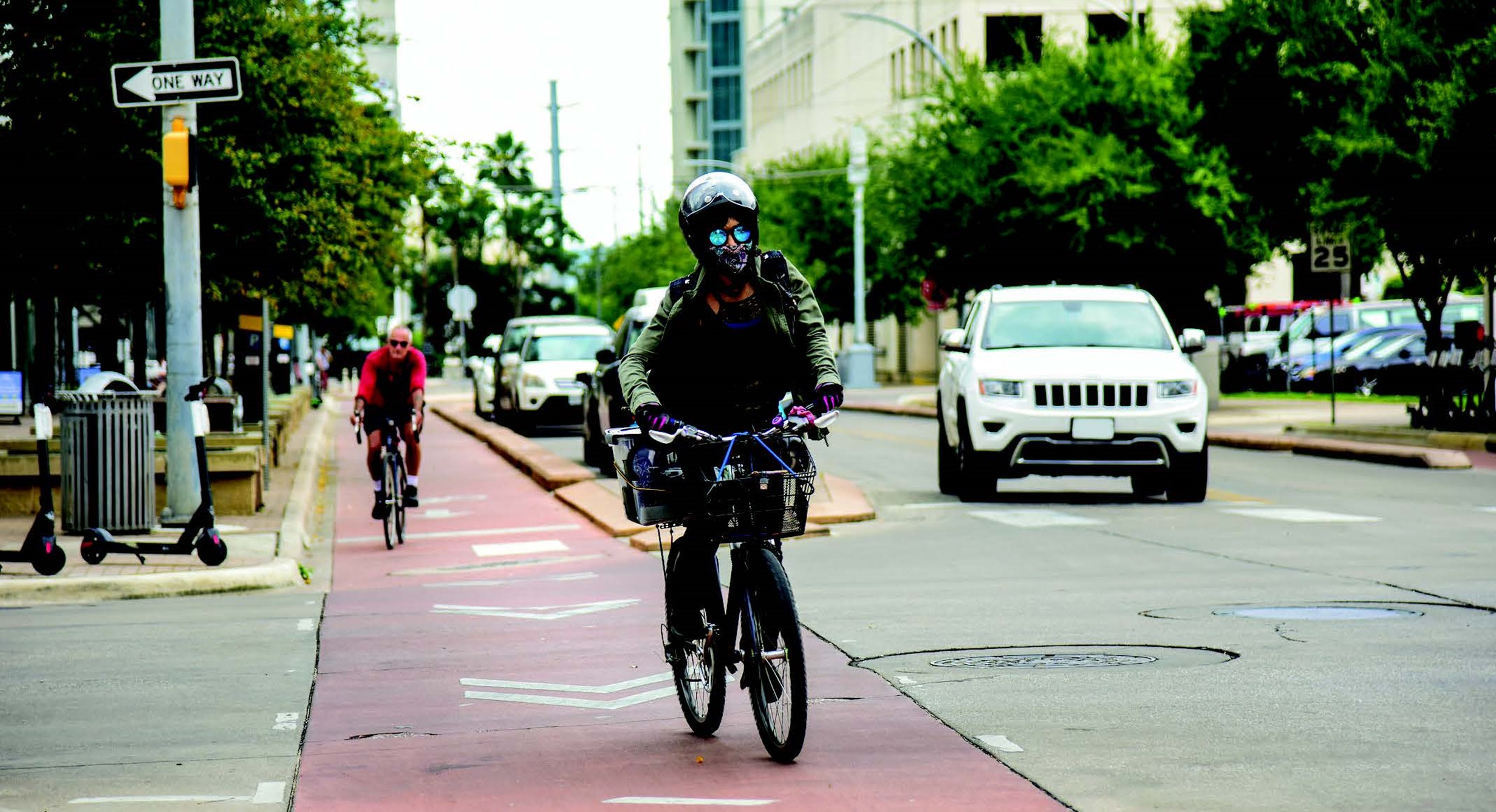
Abstract
Data-driven approaches play a critical role in developing safety improvement investment decisions. However, for non-motorized travel, exposure to risk has often been the missing piece of the puzzle. Safety analysts have been struggling with the lack of availability of exposure data, making it difficult to discern a trend in crash rates and identify high-risk locations for pedestrians and bicyclists. While short-term counts cannot be considered policy relevant (until they are scaled to a long-term representative value), continuous monitoring of non-motorized traffic using automatic sensors are often not cost effective. Moreover, every sensor has some limitations in terms of coverage, accuracy, and reliability. In the era of big data, GPS data, cell phone tracking apps, fitness tracking devices or bike sharing systems hold great potential to observe travel activity but they include a range of biases related to representation. Recognizing these limitations and benefiting from the advancements in technologies, this project aims to develop effective methodologies to fuse together different data sources to develop accurate and reliable exposure estimates for safety analysis. The proposed framework will bring together traditional and emerging data sources and will be developed in such a way that it can be up- or down-scaled based on the available data sources of a study area. The exposure estimation output will then be used for crash assessment tailored to the needs of the study area. The proposed approach will increase the quality and representativeness of data and help safety analysts effectively derive benefits from potential sources in their decision-making.
Project Highlights
- This project ventured into an emerging research territory by developing a fusion-based technique to combine multiple nonmotorized demand or exposure data using both traditional and crowdsourced data sources.
- Five bike demand models were developed to be used as inputs into the fusion framework for the selected case study area of Austin, Texas. These included the direct demand model, four-step model, bike-sharing model, Strava model, and StreetLight model.
- This research adopted the domain of decision fusion, which is an approach for combining information, where a decision from each source is classified individually to obtain a unified decision.
- Multiple decision fusion mechanisms were utilized providing an option to the analyst to utilize their knowledge of the local community and the data to either use the individual estimates or opt for the fusion approach to obtain a better estimate with increased confidence.
- The fused data were applied in both macro (hot-spot analysis at the block group level) and micro (individual safety-related perception) crash models in Austin to ascertain the significance of incorporating exposure in the safety analysis.
Final Report
EWD & T2 Products
Internship Report (pdf): A 5-week summer internship program was designed and implemented as part of this project’s EWD and T2 plan. This report describes the internship of Atom Arce, a recent high school graduate (High School for Math, Science and Engineering at CCNY – Class of 2018) and newly admitted first-year undergraduate student (Fall 2018) at the University of Toronto.
Student Impact Statement – Silvy Munira, Kyuhyun Lee, and Atom Arce (pdf): The student(s) working on this project provided an impact statement describing what the project allowed them to learn/do/practice and how it benefited their education.
Silvy Sirajum Munira served as the student researcher of the project, which constituted the core of her dissertation, entitled Fusion with Context Credibility: Exploring and Fusing Nonmotorized Traffic Data. Silvy graduated from the Civil & Environmental Engineering Department of Texas A&M University (TAMU) in summer 2021.
Kyuhyun Lee is a former student researcher/research associate at TTI. As part of the project, she examined the use and application of crowdsourced data. Kyuhyun has been accepted to a PhD degree in the Department of Urban and Regional Planning at the University of Illinois at Urbana-Champaign. She is expected to start in fall 2021.
Atom Arce is a former student intern at TTI and attended a five-week summer internship program designed/implemented in summer 2018 as part of this project. The main goal of the internship program was to provide an undergraduate student with expanded opportunities for guided learning. A detailed report was developed to describe the internship of Atom— (as of that time) a recent high school graduate (High School for Math, Science, and Engineering at CCNY—Class of 2018) and newly admitted first-year undergraduate student (Fall 2018) at the University of Toronto.
The outputs of this research are expected to be helpful to researchers, academicians, and practitioners who are looking for a methodology to bring their data together and develop analysis/models using more reliable exposure estimates.
The project team developed a 2-page visual project brief – summarizing the project and presenting the key outcomes
The research team is in developed a slide deck (as a PowerPoint presentation) that incorporates materials and knowledge gained from this project to graduate courses/seminars, such as the graduate courses taught at TAMU including Traffic Engineering, Engineering, and Urban Transportation Systems, and Seminar.
Presentations/Publications
Lee, K., & Sener, I. N. (2020). Emerging data for pedestrian and bicycle monitoring: Sources and applications. Transportation Research Interdisciplinary Perspectives, 4, 100095. (Produced as a collaborative effort from this project and another SAFE D project, entitled Data Mining to Improve Planning for Pedestrian and Bicyclists Safety)
Munira, S., & Sener, I. N. (2020). A geographically weighted regression model to examine the spatial variation of the Socioeconomic and Land-use Factors Associated with Strava Bike Activity in Austin, Texas. Journal of Transport Geography, 88, 102865.
Munira, S., Sener, I. N., & Zhang, Y. (2021). Estimating bicycle demand in the Austin, Texas Area: role of a bike ability index. Journal of Urban Planning and Development, 147(3), 04021036.
Lee, K., & Sener, I. N. (2021). Strava Metro data for bicycle monitoring: A literature review. Transport Reviews, 41(1), 27–47.
Sener, I.N. (2021) Exploring Data Fusion’s Applications to Nonmotorized Safety Analysis. Project Research Brief.
Munira, S., & Sener, I. N. (2021) Examining the spatial variation of the socioeconomic and land-use factors associated with bike activity: a case study using crowdsourced Strava data in Austin, Texas. Presented at the International Conference on Transport & Health (ICTH), Virtual Conference, June 14–30, 2021
Lee, K., & Sener, I. N. (2020). Emerging data for pedestrian and bicycle monitoring: Sources and applications. Presented at the 15th World Conference on Transport Research, Mumbai, India, May 26–31, 2019.
Munira, S., & Sener, I. N. (2020). A geographically weighted regression model to examine the spatial variation of the socioeconomic and land-use factors associated with Strava bike activity in Austin, Texas. Journal of Transport Geography, 88, 102865.
Sener, I. N., Munira, S., & Zhang, Y. (2021). Data fusion for nonmotorized safety analysis. Final Report. Safety through Disruption (Safe-D) National University Transportation Center (UTC) Program.
Final Dataset
The final datasets for this project are located in the Safe-D Collection on the VTTI Dataverse; Coming Soon.
Research Investigators (PI*)
Ipek N. Sener (TTI/TAMU)*
Silvy Munira (TTI/TAMU)
Yunlong Zhang (TAMU)
Shawn Turner (TTI-TAMU)
Kapil Arya (TTI/TAMU)
Project Information
Start Date: 06/01/2018
End Date: 07/31/2021
Status: Active
Grant Number: 69A3551747115
Total Funding: $478,437
Source Organization: Safe-D National UTC
Project Number: 03-049
Safe-D Theme Areas
Safe-D Application Areas
Planning for Safety
Vulnerable Users
More Information
Sponsor Organization
Office of the Assistant Secretary for Research and Technology
University Transportation Centers Program
Department of Transportation
Washington, DC 20590 United States
Performing Organization
Texas A&M University
Texas A&M Transportation Institute
3135 TAMU
College Station, Texas 77843-3135
USA