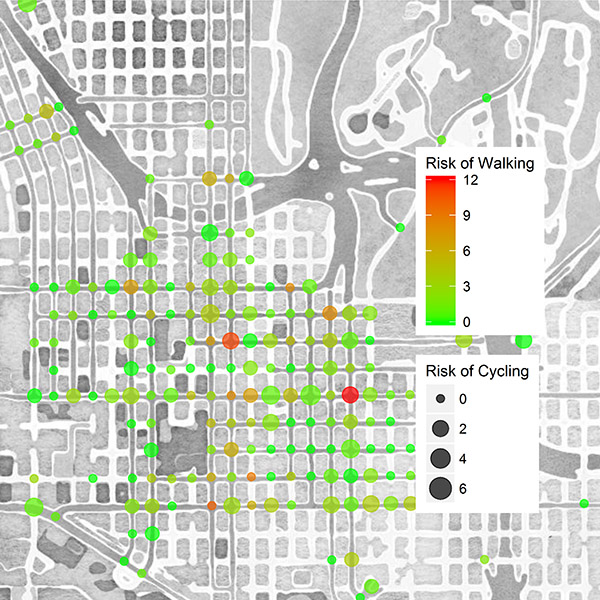
Abstract
Between 2009 and 2016, the number of pedestrian and bicyclist fatalities saw a marked trend upward. Taken together, the overall percentage of pedestrian and bicycle crashes now accounts for 18% of total roadway fatalities, up from 13% only a decade ago. Technological advancements in transportation have created unique opportunities to explore and investigate new sources of data for the purpose of improving safety planning. This study investigated data from multiple sources, including automated pedestrian and bicycle counters, video cameras, crash databases, and GPS/mobile applications, to inform bicycle and pedestrian safety improvements. Data mining techniques, a new sampling strategy, and automated video processing methods were adopted to demonstrate a holistic approach that can be applied to identify facilities with highest need of improvement. To estimate pedestrian and bicyclist counts at intersections, exposure models were developed incorporating explanatory variables from a broad spectrum of data sources. Intersection-related crashes and estimated exposure were used to quantify risk, enabling identification of high-risk signalized intersections for walking and bicycling. The modeling framework and data sources used in this study will be beneficial in conducting future analyses for other facility types, such as roadway segments, and also at more aggregate levels, such as traffic analysis zones.
Project Highlights
- Utilizing multiple data sources, such as automated pedestrian and bicycle counters and video cameras, this study estimated pedestrian and bicyclist exposure and identified signalized intersections with the highest risk for walking and bicycling.
- Exposure analysis identified transportation network, population, traffic generator, and land use variables as statistically significant in estimating pedestrian and bicyclist volume.
- The following interactive web-based tool resulted from this project, can be used to visualize high-risk intersections for walking and bicycling: https://g-hasani.shinyapps.io/RiskCalc/.
Final Report
EWD & T2 Products
Emerging Data Mining for Pedestrian and Bicyclist Monitoring: A Literature Review Report: This study reviewed currently available crowdsourced data and current use of these data. The review focuses on pedestrians and bicyclists, and includes both passive and active contributions.
Use of Direct-Demand Modeling in Estimating NonMotorized Activity: A Meta-analysis: This report reviewed various studies that used the direct-demand modeling approach to estimate pedestrian,
bicycle, and trail traffic volume at different locations. While the objectives of the studies are similar, researchers and transport planners have used a myriad of approaches based on the magnitude of the
available data and the characteristics of the study area. This report summarizes the challenges and opportunities reflected in the literature associated with the use of the direct-demand models and compares the commonalities and differences in the site-specific approaches.
Interactive Visualization Tool (link): 2. A web-based tool (Utilized Shiny, an open source R package that provides an elegant and powerful web framework for building web applications using R.) was developed to visualize high-risk intersections for walking and bicycling.
Student Impact Statements (pdf): Multiple students were funded under this project from SDSU and TTI. This file contains a statement of the impact this project made on these students’ education and workforce development.
Presentations/Publications
Sener, I.N., K. Lee, J. G. Hudson, M. Martin, B. Dai (2019) The challenge of safe and active transportation: Macrolevel examination of pedestrian and bicycle crashes in the Austin District, Journal of Transportation Safety & Security, DOI: 10.1080/19439962.2019.1645778.
Hasani, M., A. Jahangiri, I. N. Sener, S. Munira, J. M. Owens, B. Appleyard, S. Ryan, S. M. Turner and S. Ghanipoor Machiani (2019), “Identifying High-Risk Intersections for Walking and Bicycling using Multiple Data Sources in the City of San Diego”, Journal of Advanced Transportation, 2019. (Accepted)
Hasani, M., Arash Jahangiri, Sahar Ghanipoor Machiani (2019). Data Mining to Improve Planning for Pedestrian and Bicyclist. Accepted for presentation in 2019 Safe Systems Summit: Redefining Transportation Safety, Apr 23-24, 2019, Durham, NC. (Accepted)
Hasani, M., Arash Jahangiri, Ipek N. Sener, Sahar Ghanipoor Machiani (2018). Identifying High Crash Risk Intersections for Walking and Biking. Center for Human Urban Mobility (CHUM) Summit, University of California at San Diego, September 20, 2018. (Accepted)
Hasani, M. Arash Jahangiri, Sahar Ghanipoor Machiani (2018). Developing Models for Matching of Short-term and Long-term Data Collection Sites to Improve the Estimation of Average Annual Daily Bicyclists. The 21st IEEE International Conference on Intelligent Transportation Systems. November 4-7, 2018, Maui, Hawaii, USA (Published)
Hasani, M., Jahangiri, A. Utilizing extrapolation methods to estimate average annual daily bicyclist and pedestrian volume from short-term count data in the City of San Diego, CA. Student Research Symposium, San Diego State University, March 2, 2018. (Published)
Zhang, C., Jahangiri, A. Adopting cumulative learning to develop a real-time vision-based monitoring system for detecting and tracking vulnerable road users. Student Research Symposium, San Diego State University, March 2, 2018. (Published)
Lee, K., and I.N. Sener. “Emerging Data Mining for Pedestrian and Bicyclist Monitoring: A Literature Review Report”, Technical Report, prepared for the Safety through Disruption (Safe-D) National University Transportation Center (UTC) Program, September 2017. Accepted for presentation in 15th World Conference on Transport Research, 26-31 May 2019, Mumbai, India
Sener, I.N., K. Lee, J.G. Hudson, M. Martin, B. Dai (2018). “The Challenge of Safe and Active Transportation: Examination of Pedestrian and Bicycle Crashes in the Austin District”. Technical Paper prepared for Texas Department of Transportation Austin District and Safe-D UTC Program. Presented at the 98th Annual TRB Meeting, January 13–17, 2019, Washington, DC.
Research Investigators (PI*)
Arash Jahangiri (SDSU)*
Sherry Ryan (SDSU)
Bruce Appleyard (SDSU)
Ipek N. Sener (TTI/TAMU)
Shawn Turner (TTI/TAMU)
Justin Owens (VTTI/VT)
Project Information
Start Date: 2017-04-01
End Date: 2018-12-30
Status: Complete
Grant Number: 69A3551747115
Total Dollars: $289,793
Source Organization: Safe-D National UTC
Project Number: 01-003
Safe-D Theme Areas
Safe-D Application Areas
Risk Assessment
Vulnerable Users
Planning for Safety
More Information
Sponsor Organization
Office of the Assistant Secretary for Research and Technology
University Transportation Centers Program
Department of Transportation
Washington, DC 20590 United States
Performing Organization
San Diego State University
5500 Campanile Dr
San Diego, CA 92182
USA
Texas A&M University
Texas A&M Transportation Institute
3135 TAMU
College Station, Texas 77843-3135
USA
Virginia Polytechnic Institute and State University
Virginia Tech Transportation Institute
3500 Transportation Research Plaza
Blacksburg, Virginia 24061
USA