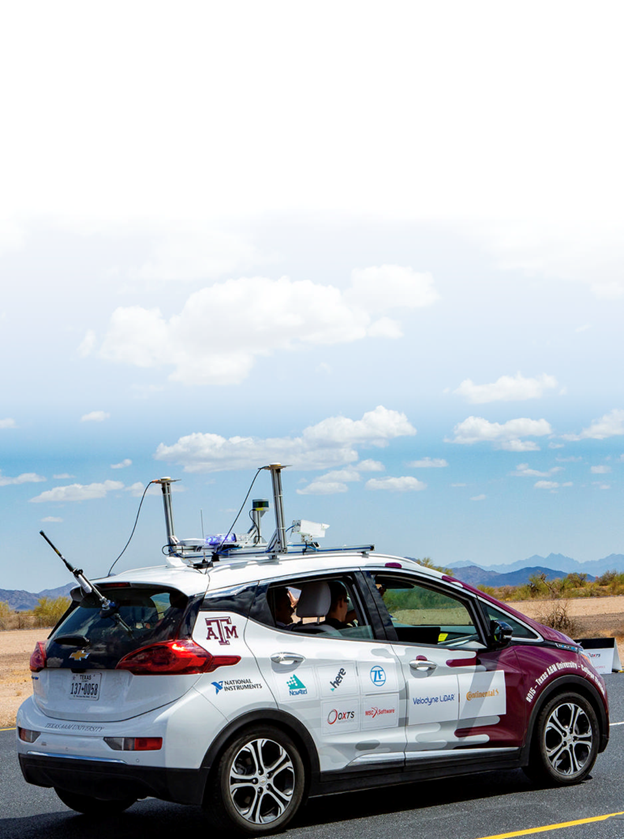
Abstract
While safety is the ultimate goal of designing connected automated vehicles (CAVs), in many instances, CAVs’ decisions do not match the expectations of human drivers (e.g., 3-second stopping rules versus rolling stops performed by human drivers). Such instances can lead to crashes/near-crashes; for instance, in 18 out of 26 crashes involving CAVs in California through February 2017, a CAV was rear-ended by a human driver at an intersection. Unfortunately, the state-of-the-art in CAV safety analysis is focused on actual and simulated miles driven, which are shown to be infeasible to apply after each update (software and/or hardware). Accordingly, this project is expected to bring insight from traffic safety analysis to develop a systematic approach for CAV safety evaluation. This project will identify the factors that contribute to crashes in mixed traffic with automated and human-driven vehicles through data analysis, simulation, and field tests. Moreover, it will develop measures and guidelines to minimize the risk of such crashes. The findings of this study are expected to significantly enhance the safety of operating CAVs.
Project Highlights
- Identified a novel near-crash risk estimation technique to accurately predict 84% of the potential rear-end near-crashes in the programming language Python
- Modeled potential near-crash and safe driving events using Random Forest classification algorithm (RStudio, Tableau, Excel)
- Estimated Autonomous Vehicle near-crash risk with human-driven vehicles using popular forward-collision warning algorithms
Final Report
EWD & T2 Products
Master’s Thesis, Aman Sharma December 2019, Texas A&M University, M.S. Civil Engineering. Thesis title: Analyzing Crash Potential in Mixed Traffic with Autonomous and Human-Driven Vehicles
Student Impact Statement – Aman Sharma (pdf): The student(s) working on this project provided an impact statement describing what the project allowed them to learn/do/practice and how it benefited their education.
Presentations/Publications
Rahmati, Y., Khajeh Hosseini, M., Talebpour, A., Swain, B., & Nelson, C. (2019). Influence of autonomous vehicles on car-following behavior of Human drivers. Transportation Research Record, 2673(12), 367-379.
Rahmati, Y., A., Samimi Abianeh, M., Tabesh, A., Talebpour, and F., Sharifi. (2019) Driving to Safety: Who Is at Fault in CAVs Rear-End Collisions. Presented at the 98th Annual Meeting of the Transportation Research Board of National Academies, January 13-17, 2019.
Rahmati, Y., A., Samimi Abianeh, M., Tabesh, A., Talebpour, and F., Sharifi. Driving to Safety: Who Is At Fault in CAVs Rear-End Collisions. Submitted for Publications in Transportation Research Part A.
Datasets
The final datasets for this project is unavailable due to the departure due to the departure of the PI and student in December 2019.
Alireza Talebpour (TTI/TAMU)*
Dominique Lord (TTI/TAMU)
Michael Manser (TTI/TAMU)
Sahar Ghanipoor Machiani (SDSU)
Project Information
Start Date: 05/01/2018
End Date: 12/31/2019
Status: Complete
Grant Number: 69A3551747115
Total Funding: $172,648
Source Organization: Safe-D National UTC
Project Number: 03-072
Safe-D Theme Areas
Automated Vehicles
Connected Vehicles
Safe-D Application Areas
Risk Assessment
Driver Factors and Influences
Planning for Safety
More Information
Sponsor Organization
Office of the Assistant Secretary for Research and Technology
University Transportation Centers Program
Department of Transportation
Washington, DC 20590 United States
Performing Organization
Texas A&M University
Texas A&M Transportation Institute
3135 TAMU
College Station, Texas 77843-3135
USA
San Diego State University
5500 Campanile Dr
San Diego, CA 92182
USA