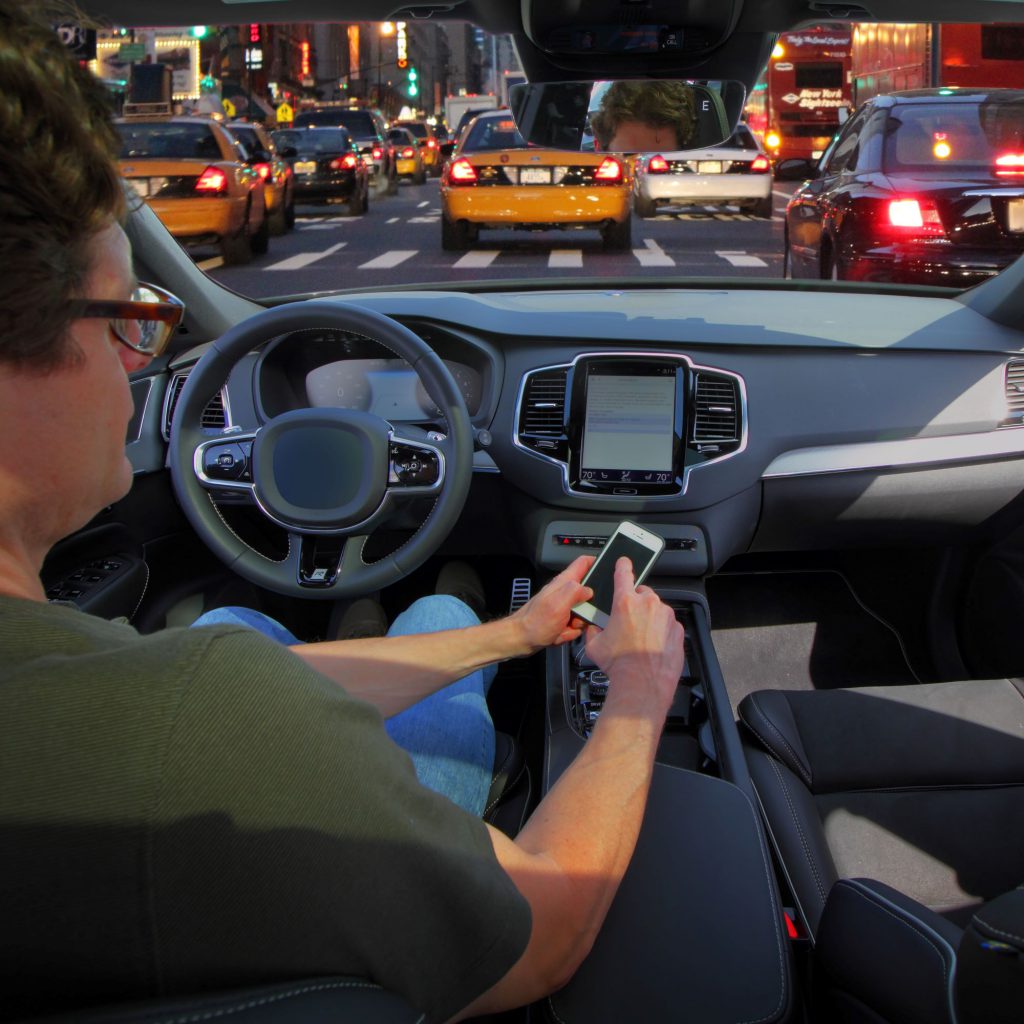
Abstract
This project addressed the emerging field of behavior-based predictive safety analytics, focusing on the prediction of road crash involvement based on individual driver behavior characteristics. This has a range of applications in the areas of fleet safety management and insurance, but may also be used to evaluate the potential safety benefits of an automated driving systems. This project continued work from a pilot study that created a proof-of-concept demonstration of how crash involvement may be predicted on the basis of individual driver behavior utilizing naturalistic data from the Second Strategic Highway Research Program. The current project largely focuses on understanding and identifying the risks from a driver based on their driving behaviors, personal characteristics, and environmental influences. This project analyzed large scale continuous naturalistic data as well as event data to study the role of different driving behaviors in the buildup of risk related to a safety-critical event or crash. This research can be used to structure the development of real-time crash risk that accounts for those identified driver behaviors to be evaluated across the contextualized information on a roadway.
Project Highlights
- The ability to classify drivers according to their driving style, as defined by actual behaviors on road, leads to increasingly accurate identification of risky drivers and risky behaviors.
- Several recommendations emphasize certain next steps for research and industry to undertake effective evaluation of real-time crash risk.
- The project provides justification for the collection of trip-level data across the industry as well as the collection of individual characteristics that will influence a driver’s propensity for being involved in crashes. This effort is necessary to understand the capacity and capabilities for ongoing worldwide zero-fatality efforts.
Final Report
EWD & T2 Products
Student Impact Statement(pdf): Four graduate students worked on this project (Andrei Svetovidov, a Master’s student in Computer Science, Zehou (Jerry) Dong, a Master’s student in Industrial and Systems Engineering, Ioannis Papakis, a PhD student in Mechanical Engineering and Daniel Ik-Obazee, a Master’s student in Industrial and Systems Engineering, all from Virginia Tech University). This file contains a statement by Andrei Svetovidov as to the impact this project had on education and workforce development.
Education module developed for an Undergraduate Psychology, Research Methods, Human Factors, Injury Biomechanics, Industrial Systems Engineering, or other accident theory-oriented course. Course Material is available here.
Presentations/Publications
Coming Soon!
Research Investigators (PI*)
Andrew Miller (VTTI)*
Abhijit Sarkar (VTTI)
Tony McDonald (TTI/TAMU)
Sahar Ghanipoor-Machiani (SDSU)
Arash Jahangiri (SDSU)
Project Information
Start Date: 2019-03-01
End Date: 2022-11-30
Status: Complete
Grant Number: 69A3551747115
Total Funding: $191,534
Source Organization: Safe-D National UTC
Project Number: 04-114
Safe-D Theme Areas
Safe-D Application Areas
Risk Assessment
Driver Factors and Interfaces
Vehicle Technology
Freight and Heavy Vehicles
Performance Measures
More Information
Sponsor Organization
Office of the Assistant Secretary for Research and Technology
University Transportation Centers Program
Department of Transportation
Washington, DC 20590 United States
Performing Organization
Virginia Polytechnic Institute and State University
Virginia Tech Transportation Institute
3500 Transportation Research Plaza
Blacksburg, Virginia 24061
USA
Texas A&M University
Texas A&M Transportation Institute
3135 TAMU
College Station, Texas 77843-3135
USA
San Diego State University
5500 Campanile Dr
San Diego, CA 92182
USA