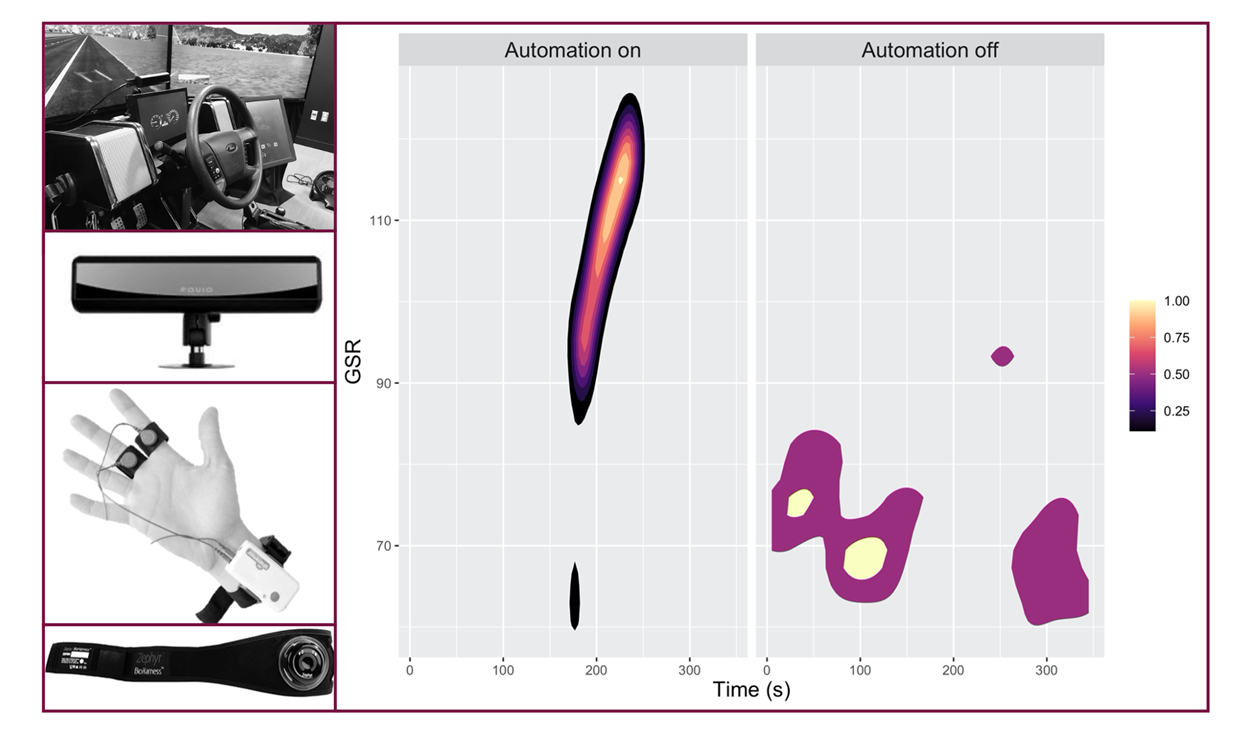
Abstract
Advanced driver assistance systems (ADAS) have significantly improved safety on today’s roadways but their impact may be limited by driver errors. Understanding and identifying these driver errors will require the integration of multi-domain datasets through predictive modeling and data integration approaches. The goals of this project are to identify relevant datasets for ADAS error prediction, evaluate modeling approaches for predicting driver errors during ADAS use, and developing models to proactively predict driver errors. Results from the project will be used to guide data collection system design at automakers and develop predictive modeling benchmarks.
Project Highlights
- The project resulted in the validation of modeling techniques for predicting driver behaviors.
- The project provided direct training to a PhD level student and resulted in a TRB conference presentation.
Final Report
EWD & T2 Products
Student Impact Statement – Hananeh Alambeigi (pdf): The student(s) working on this project provided an impact statement describing what the project allowed them to learn/do/practice and how it benefited their education.
Presentations/Publications
Alambeigi, H. , McDonald, A.D., Manser, M., Shipp, E., Lenneman, J., Pulver, E., Christensen, S. (2021). Predicting Driver Errors During Automated Vehicle Takeovers. Transportation Research Record. In Revision.
Alambeigi, H., McDonald, T., Shipp, E., & Manser, M. (2021). Identifying deviations from normal driving behavior. TTI-Student-08
McDonald, A.D. & Alambeigi, H. (2021). Investigating the effects of silent automation failure and scenario criticality on automated vehicle’s take-over performance. US DOT SAFE-D National Transportation Research Center https://doi.org/10.15787/VTT1/C76VBC
Final Dataset
The project’s used a dataset from a prior Safe-D project 03-036. The final datasets for this project are located in the Safe-D Collection on the VTTI Dataverse; DOI: 10.15787/VTT1/C76VB.
Research Investigators (PI*)
Michael Manser (TTI/TAMU)*
Tony McDonald (TTI/TAMU)*
Eva Shipp (TTI/TAMU)*
Hananeh Alambeigi (TTI/TAMU)
Project Information
Start Date: 2020-10-20
End Date: 2021-10-15
Status: Completed
Grant Number: 69A3551747115
Total Funding: $49,493
Source Organization: Safe-D National UTC
Project Number: TTI-Student-08
Safe-D Theme Areas
Big Data Analytics
Automated Vehicles
Safe-D Application Areas
Performance Measures
Vehicle Technology
More Information
RiP URL
UTC Project Information Form
Sponsor Organization
Office of the Assistant Secretary for Research and Technology
University Transportation Centers Program
Department of Transportation
Washington, DC 20590 United States
Performing Organization
Texas A&M University
Texas A&M Transportation Institute
3135 TAMU
College Station, Texas 77843-3135
USA
Image Collected from Freight Wave, & Prevost, C. (2018, May 7). [Semi-Trucks congested in a dense parking lot.]. What Is the Solution to Trucking’s Stubborn Parking Problem? https://www.freightwaves.com/news/driver-issues/what-is-the-solution-to-truckings-stubborn-parking-problem