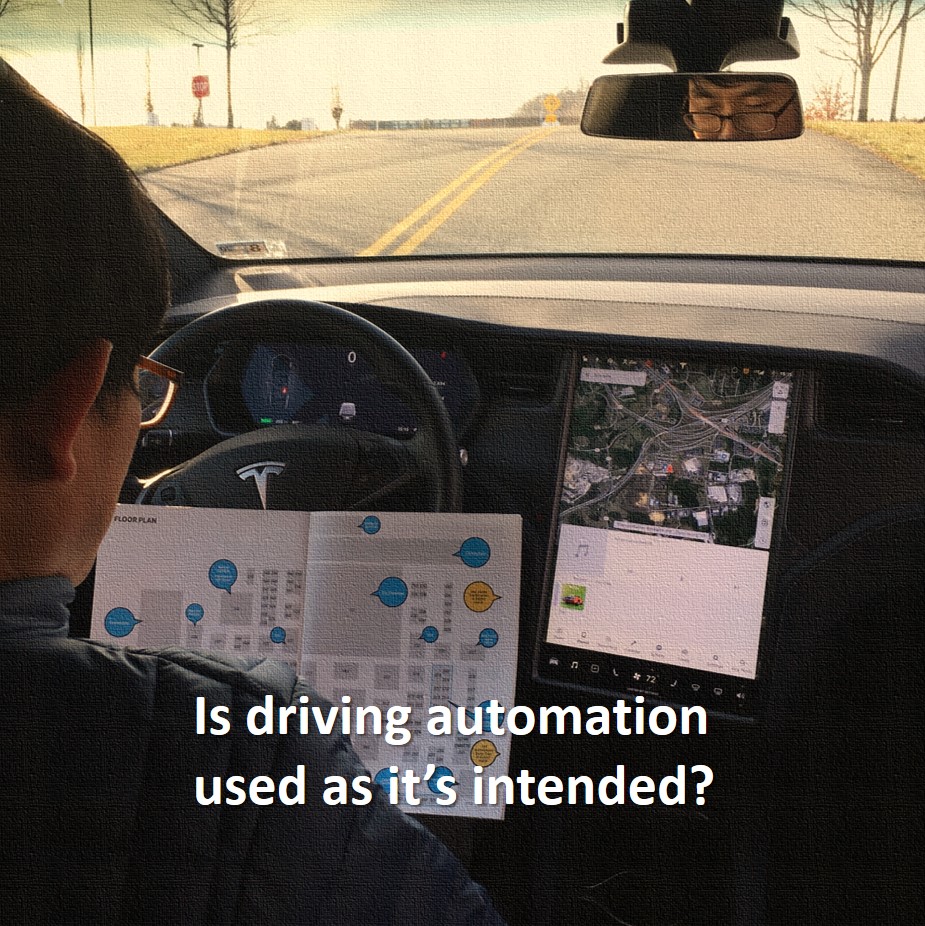
Abstract
Automated driving systems (ADSs) have the potential to fundamentally transform transportation by reducing crashes, congestion, and cost while improving traffic efficiency and access to mobility for the transportation-challenged population. However, people may not use ADS as intended due to their misunderstanding of such systems’ capabilities and limitations. Recent news articles, reporting Tesla drivers napping behind the wheel, suggest the need for a better understanding of how people are using ADSs as well as what benefits and consequence that such systems have on transportation safety. Therefore, this work aims to investigate the (1) limitations of automated longitudinal and lateral control features (e.g., adaptive cruise control and lane keeping assistance) found in real-world operation, (2) unintended use of such systems and their safety consequences, as well as (3) driver perception of these novel technologies. For this purpose, this study will leverage data collected from 50 participants who drove personally owned vehicles equipped with ADSs for 12 months. The work is expected to contribute to a greater understanding of the prevalence and safety consequences of ADS use on public roadways, as well as drivers’ perception of the early production ADS. The findings from this project may further inform the development of human-machine interfaces, training programs, and owners’ manuals to reduce unintended use of ADSs and negative consequences. The identified characteristics of the situations when the driving automation requested human drivers’ intervention or failed without alerts will further inform the development of testing scenarios to ensure ADS safety.
Project Highlights
- An in-depth analysis of the naturalistic driving study (NDS) dataset revealed that 47 out of 235 (20%) safety-critical events (SCEs) involved an automated driving system (ADS) use. An in-depth analysis of 47 SCEs revealed that people misused ADSs in 57% of SCEs (e.g., engaged in secondary tasks, used the systems not on highways, or with hands off the wheel).
- During 13% of SCEs, the ADSs neither reacted to the situation nor warned the driver. These instances may be perceived as silent failures which drivers many not expect.
- A post-study survey showed that people found ADSs useful and usable. However, the more participants were positive to ADS features, the more they felt comfortable engaging in secondary tasks, which is an unintended side effect of Level 2 ADSs as they require the human driver’s supervision.
- Participants reported that the longitudinal control features did not respond well to cutting-in leads (23% of participants) and stopped leads (14%). The lateral control features were often automatically disengaged when encountering blurred lane markings (14% of participants reported) and had difficulties when negotiating curves (9%).
- The research methods and findings have been translated into a graduate-level learning module entitled “Naturalistic Driving Studies for Automated Driving Systems”.
Final Report
EWD & T2 Products
Research Findings & Lessons Learned (pptx): These slides provide an overview of the project along with methods, results, findings, implications, and lessons learned on how to improve upon the research.
Course Module (pptx): This presentation is a course module developed from this project to introduce students to the project and the dataset.
Student Impact Statement – Adam Novotny (pdf) and Rick Greatbatch (pdf): The student(s) working on this project provided an impact statement describing what the project allowed them to learn/do/practice and how it benefited their education.
Presentations/Publications
Kim, H., Miao Song, & Doerzaph, Z. (2020). Real-World Use of Partially Automated Driving Systems and Driver Impressions. In Proceedings of the Human Factors and Ergonomics Society Annual Meeting (Presented), Sage CA: Los Angeles, CA: SAGE Publications.
Yang, L., Furukawa, T., Zuo, L., Parker, R., & Doerzaph, Z. (2019). Level-of-Confidence Driven Automatic Emergency Stop to a Safe Roadside. 5th International Symposium on Future Active Safety Technology toward Zero Accidents (FAST-zero-19). Blacksburg, VA.
A manuscript: Kim, H., Miao Song, & Doerzaph, Z. (2020). Real-World Use of Partially Automated Driving Systems and Driver Impressions. In Proceedings of the Human Factors and Ergonomics Society Annual Meeting (Accepted)
Final Dataset
The final datasets for this project are located in the Safe-D Collection on the VTTI Dataverse; DOI: 10.15787/VTT1/98NBN7.
Research Investigators (PI*)
Hyungil Kim (VTTI)*
Miao Song (VTTI/VT)
Rick Greatbatch (VT-Student)
Adam Novotny (VTTI/VT)
Zac Doerzaph (VTTI)
Project Information
Start Date: 2019-11-01
End Date: 2020-07-30
Status: Complete
Grant Number: 69A3551747115
Total Funding: $80,326.00
Source Organization: Safe-D National UTC
Project Number: VTTI-00-029
Safe-D Theme Areas
Automated Vehicles
Big Data Analytics
Safe-D Application Areas
Risk Assessment
Planning for Safety
Operations and Design
Vehicle Technology
More Information
Sponsor Organization
Office of the Assistant Secretary for Research and Technology
University Transportation Centers Program
Department of Transportation
Washington, DC 20590 United States
Performing Organization
Virginia Polytechnic Institute and State University
Virginia Tech Transportation Institute
3500 Transportation Research Plaza
Blacksburg, Virginia 24061
USA