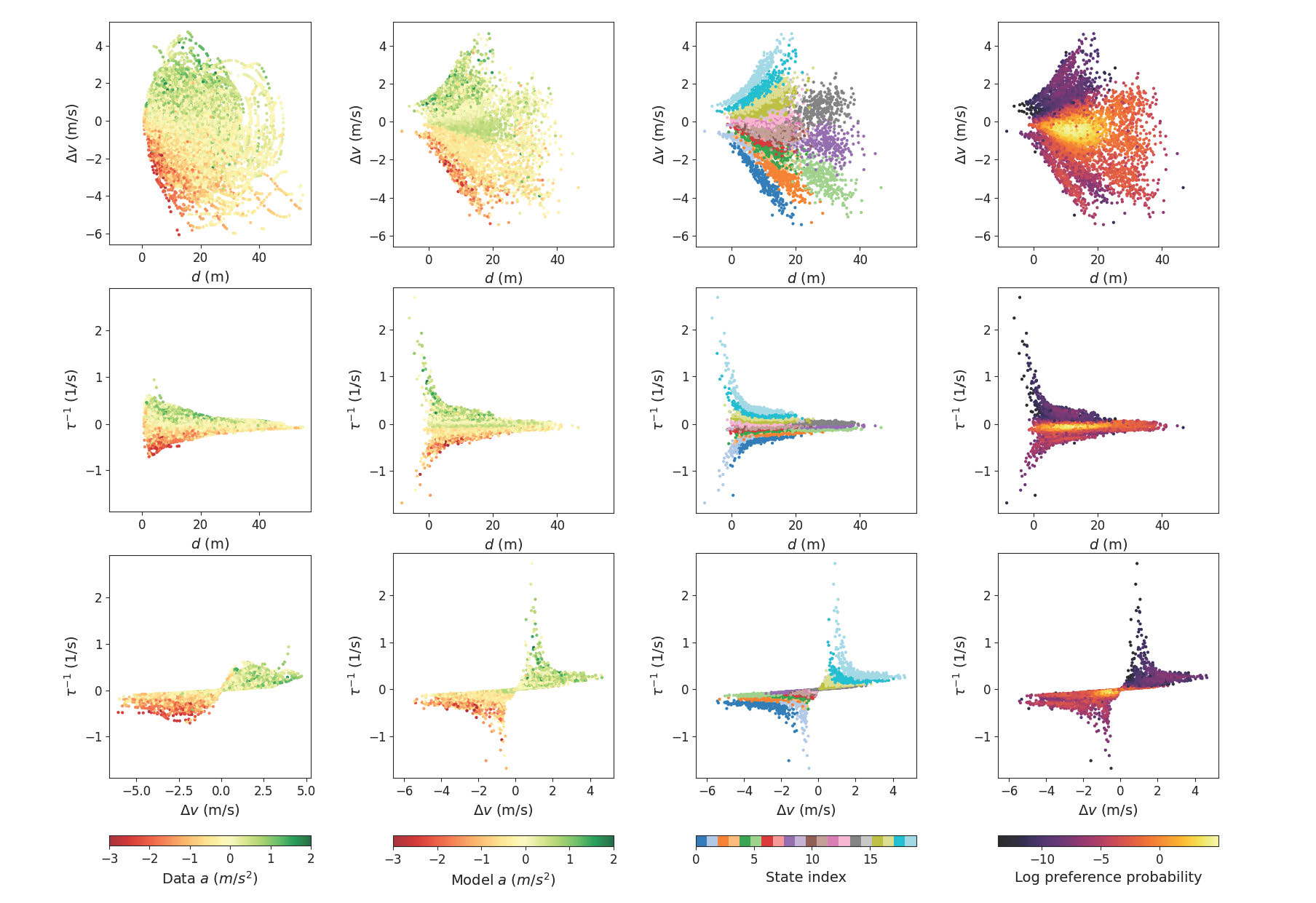
Abstract
Driver process models play a central role in the testing, verification, and development of automated and autonomous vehicle technologies. Prior models developed from control theory and physics-based rules are limited in automated vehicle applications due to their restricted behavioral repertoire. Data-driven machine learning models are more capable than rule-based models but are limited by the need for large training datasets and their lack of interpretability. In this project we developed a novel car following modeling approach using active inference, which has comparable behavioral flexibility to data-driven models while maintaining interpretability. We assessed the proposed model, the Active Inference Driving Agent (AIDA), through a benchmark analysis against several benchmarks. The models were trained and tested on a real-world driving dataset using a consistent process. The testing results showed that the AIDA predicted driving controls significantly better than the rule-based Intelligent Driver Model and had similar accuracy to the data-driven neural network models in three out of four evaluations. Subsequent interpretability analyses illustrated that the AIDA’s learned distributions were consistent with driver behavior theory and that visualizations of the distributions could be used to directly comprehend the model’s decision-making process and correct model errors attributable to limited training data.
Project Highlights
- Statistically accurate and interpretable models of human perception and action in driving related tasks has enormous implications for the successful deployment of autonomy. This is because such models facilitate the design and real-time execution of the interaction between autonomous vehicles and humans.
Final Report
EWD & T2 Products
Wei R., McDonald A. D., Garcia A. and Alambeigi H. (2022) Modeling Driver Responses to Automation Failures With Active Inference, IEEE Transactions on Intelligent Transportation Systems 23 (10) 18064-18075, doi: 10.1109/TITS.2022.3155381.
Wei R., Garcia, A., McDonald A.D., Markkula G., Engstrom J., Supeene I. and O’Kelly M. (2022). World Model Learning From Demonstrations With Active Inference: Application to Driving Behavior, 3rd International Workshop on Active Inference (IWAI).
Wei R., McDonald A.D., Garcia, A., Markkula G., Engstrom J. and O’Kelly M., (2023). An active inference model of car following: Advantages and applications, doi: 10.48550/arXiv.2303.15201
Wei R., Zeng S., Li Ch., Garcia A., McDonald A. and Hong M. (2023) Robust Inverse Reinforcement Learning Through Bayesian Theory of Mind, ICML 2023 Workshop on Theory of Mind in Communicating Agents
Developed a software package for implementing active inference models of driver behavior and released the software via GitHub. https://github.com/rw422scarlet/interactive_inference
Wei, Ran. (June, 2023). Learning representations of cognitive dynamics and decision making in human drivers. [Doctoral Dissertation, Texas A &M University).
Developed lecture material introduction to predictive processing for the course ISyE 516 “Introduction to Decision Making”. Lecture available here.
Presentations/Publications
Garcia, A. (2023, September 20). Learning Active Inference Models of Perception and Control. Webinar SafeD Virtual Webinar is available here. PDF of PowerPoint slides from Webinar available here.
Research Investigators (PI*)
Project Information
Start Date: 2022-01-15
End Date: 2023-06-30
Status: Complete
Grant Number: 69A3551747115
Total Funding: $150,000
Source Organization: Safe-D National UTC
Project Number: 06-009
Safe-D Theme Areas
Automated Vehicles
Big Data Analytics
Safe-D Application Areas
Driver Factors and Interfaces
Planning for Safety
Vehicle Technology
Performance Measures
Operations and Design
More Information
UTC Project Information Form
Sponsor Organization
Office of the Assistant Secretary for Research and Technology
University Transportation Centers Program
Department of Transportation
Washington, DC 20590 United States
Performing Organization
Texas A&M University
Texas A&M Transportation Institute
3135 TAMU
College Station, Texas 77843-3135
USA